Data governance is an essential but often under-supported topic when researching and considering purchases in the Internet of Things (IoT).
You often see references to the multiple “layers” of IoT: the physical infrastructure, network security, integration with your existing software and data analytics are all essential parts of an effective deployment.
But what’s often glossed over, or missed entirely, is the “people layer” of IoT – how you and your employees interact with this technology and how you must structure rules and responsibilities to use the data you collect effectively.
In fact, it’s not difficult to find information about IoT data waste, sometimes referred to as digital waste. Data that is discarded or that languishes in storage because no one has decided what to do with it.
And while we’re not going go into too much depth on this point, data storage, particularly cloud data storage, is energy intensive. Figures vary slightly on this, but as much as 0.2 tons of CO2 is produced for every 100GB of data stored.
There’s no authoritative figure on how much IoT data is wasted, but most estimations we could find suggest an average between 50-99%, which, if reliable, is very high given the capital investment necessary to buy and deploy most IoT systems.
This outcome can be avoided with appropriate IoT data governance. This article explains what IoT data governance is, why it’s critical to your success with this technology and a basic overview of how to implement it.
The result should be greater control over your data assets while getting the best value possible from your IoT investment.
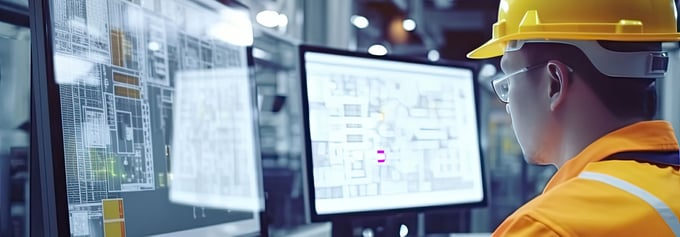
What is IoT data governance, and why does it matter?
A lot of the challenge of getting the full value out of IoT is getting the people involved to have a shared understanding of that value, what the data it creates is for, how it can be used and who is responsible for it.
Effective governance and implementation of the Internet of Things require a long view of the issues and problems an organization is trying to solve. IoT systems alone won’t solve inefficiencies or provide insights into new opportunities – they only work as part of a long-term approach to these issues.
Data governance is then about supporting the connection between the data and an organization’s business objectives, whether that’s reducing costs, improving employee performance, or automating processes.
You are almost certainly already doing some kind of data governance as part of your normal business operations. But if your approach to data governance right now is to react when a problem arises and then repeat the same fix every time it reoccurs, you’ll likely find it impossible to gain significant efficiencies from implementing IoT technology.
To succeed, you need to think about IoT data as a way of preventing problems rather than only as a tool for resolving them when they happen.
This also means you need to get specific about what problems you want to solve.
To give an example – the Severn WLD water leak detection system we make here at LAIIER is designed with a focus on regular water leaks in commercial spaces that frequently happen in hidden or hard-to-reach places.
Say you deployed our sensors in an apartment building where facilities management spends a large chunk of their time repairing or following up on water leaks in the units.
It’s normal for leaks to crop up in the units from time to time, but it’s impossible to know where the next one will happen.
Suppose that leaks are beginning to use up an inordinate amount of maintenance hours. In some cases, leaks are only discovered when tenants can see the water coming through the drywall in the unit below – having already done a lot of damage.
Crucially, because maintenance is so busy, they don’t have time to record the particular details of every incident they get to every day, so it’s difficult to see any larger patterns in where leaks are happening or how repairs are being made.
When deployed, our sensors alert facilities management to tiny water leaks, meaning they know about them before they have caused damage.
And every time a tiny leak occurs, that gets recorded as data. Over time, this dataset is analyzed and used to predict where leaks are likely to occur next.
You can now begin to shift the work your maintenance staff is doing. Instead of running around trying to save units from major water damage, they are proactively maintaining plumbing and infrastructure.
That is an ideal outcome, but achieving that requires a solid foundation of data governance that facilitates this transformation. Sadly, that doesn’t come in the box with the sensors.
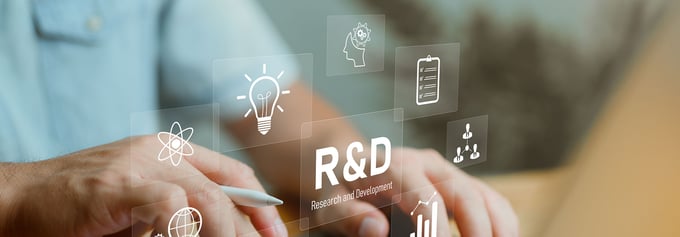
How to get started with IoT data governance
Data governance isn’t as complicated as it’s sometimes made out to be – data management professionals use a lot of technical terms that, while very precise, are challenging to get your head around if you’re not a specialist yourself.
The following is a common process used to get started with data governance and help you figure out what you need to effectively manage the use of your IoT-generated data.
Depending on what IoT tech you use, this may take time and can get complex, but without it, you’re potentially missing out on the value you should be receiving.
Step 1: Take stock of your assets
The first thing to do is to take stock of what data assets you have, where it all comes from and where you can access it right now. This should include data you gather from non-IoT sources as well.
Again, to use the example of water leaks, this might include data from sensors that detect leaks, flow, or pressure. It might also include reports from tenants and your maintenance staff.
It could also include information you have about the infrastructure itself: records of the dishwashers and washing machines in each unit and all the pipes, valves, fittings and drains throughout the building.
As part of this process, you may even discover you have assets you didn’t know you had while taking a hard look at the ones you knew about. This tends often happens because before now, no one thought to ask if it existed or what value it could have.
Step 2: Categorize your data
To make sense of all the assets you’ve collected, your data needs to be organized.
The key issue is that it is all structured in some kind of logical way. For instance, it is common at this stage to assign everything to a category: input data from your maintenance staff as one category, product data about appliances in another – it’s important that whatever structure you choose, it makes sense to you.
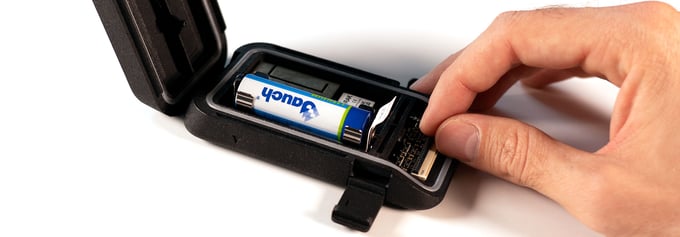
Step 3: Create policies and rules
Now comes the part where you need to make decisions about your data assets.
Creating policies for IoT data starts with deciding what data you want to keep. While we noted earlier that businesses discard more than half of the IoT data they collect, you should expect that some of the data is not going to be useful in meeting your business goals.
You may even want a rule that specifies that data of a certain type or that fails to meet specific criteria be discarded because it just produces unnecessary clutter and waste.
This is also the stage to consider compliance. There are laws and regulations around data collection and usage you must follow, depending on your jurisdiction. Many data collection regimes are specific about how you must store and secure your data – you should create policies and rules to cover these requirements.
Finally, you should be defining who is responsible for data collection, who within your organization has access to different kinds of data, and what their responsibilities are surrounding that access.
To use the example again, you probably want maintenance staff to have access to alerts from your water sensors so they are informed immediately of any leaks. Meanwhile, your IT staff has access to aggregated, anonymized data they can use to generate reports and run analyses on.
Step 4: Label your IoT data
You have your data assets, you’ve organized them and built a structure of rules and policies around them. To make use of the algorithmic analysis and machine learning tools, your data needs to be labeled.
How data is labeled is almost certainly going to be in the context of a conversation you’re having either with your IT team or an IT vendor, but you can think of it a little bit like putting all your data into boxes and then writing on the side of each box what it contains.
This might include labeling each type of data you have, when it was collected, how it was collected, and importantly, what its relative business value is for your organization.
Step 5: Automate
This is essentially the end result of good IoT data governance. If done well, you should find yourself knowing where all your data is, how it is being collected, handled and stored, and what value it is supposed to deliver.
This is the step where you start using analysis, often with machine learning tools, and start looking for patterns and developing a wider vision that the Internet of Things can show you: where to find efficiencies, reduce costs, and shift the work of your organization from a reactive stance when it comes to dealing with problems to a more proactive one.
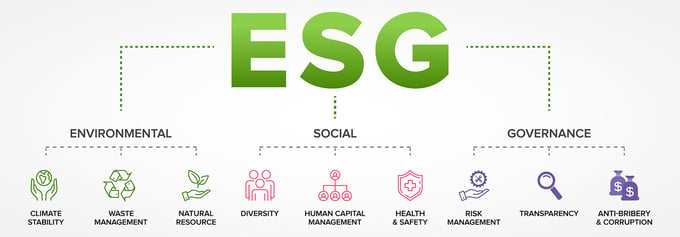
Check out more content on managing IoT for your organization
IoT data management is an underserved element in the process of putting the power of this technology to work for businesses. We published how the use of IoT, and its management, can support your organization’s Environmental Social Governance (ESG) objectives and make your brand more attractive to today’s investors.